Table of Contents
- Introduction
- The Evolution of Business Data Analytics
- Quality Data vs. Big Data: Understanding the Difference
- The Rise of In-House Analytics Capabilities
- Adapting to Changing Client Needs
- The Growing Importance of Soft Skills in Data Analytics
- Breaking Down Organizational Silos with Analytics
- The Role of AI and Machine Learning in Modern Analytics
- Addressing Privacy Concerns in Data Collection
- Future Trends in Customer Analytics
- Conclusion
- FAQ
Introduction
Imagine you’re swimming in an ocean of information. That’s what many businesses feel like these days when it comes to data. It’s everywhere, and there’s more of it every second. Pretty exciting, right? Well, yes and no. You see, companies are waking up to the fact that data isn’t just nice to have – it’s essential for making smart decisions. But here’s the catch: not all data is created equal. It’s like trying to find a few golden nuggets in a river full of pebbles. The challenge isn’t just having data anymore; it’s figuring out which data actually matters. That’s where things get interesting. Professional data wizards (okay, we call them business data analytics firms) are changing how they work. They’re not just number crunchers anymore. These folks are becoming guides, helping companies navigate this data flood and fish out the good stuff – the insights that can really make a difference.
In this article, we’re going to take a closer look at how these data pros are adapting to this new world. We’ll explore how they’re helping businesses not just collect data, but make sense of it all. It’s a bit like turning a jumble of puzzle pieces into a clear picture. Intrigued? Let’s dive in and see how companies are learning to swim in this sea of data, and maybe pick up a few tips ourselves along the way.
The Evolution of Business Data Analytics
From Data Scarcity to Data Abundance
The field of business data analytics has undergone a remarkable transformation in recent years. What was once a landscape characterized by data scarcity has now become one of data abundance. This shift has brought both opportunities and challenges for organizations seeking to leverage data for competitive advantage.
Key Milestones in Business Analytics Evolution
Era | Characteristic | Focus |
---|---|---|
1990s | Data Warehousing | Centralized data storage |
2000s | Business Intelligence | Reporting and dashboards |
2010s | Big Data | Volume, velocity, variety |
2020s | Quality Insights | Actionable, predictive analytics |
As we move further into the 2020s, the focus has shifted from simply accumulating large volumes of data to extracting quality insights that can drive tangible business outcomes.
Quality Data vs. Big Data: Understanding the Difference
Defining Quality Data
Quality data refers to information that is:
- Accurate and reliable
- Relevant to business objectives
- Timely and up-to-date
- Consistent across different sources
- Complete and comprehensive
The Pitfalls of Big Data Obsession
While big data has been a buzzword for years, many organizations have fallen into the trap of collecting vast amounts of data without a clear strategy for utilization. As Eric Bradlow, co-founder of the Wharton Customer Analytics Initiative (WCAI), notes:
“Much of the data collected by companies is not predictive and is, in fact, useless.”
This realization has led to a paradigm shift in how businesses approach data collection and analysis.
The Rise of In-House Analytics Capabilities
Factors Driving In-House Analytics Adoption
- Increased accessibility of analytics tools
- Growing data literacy among business professionals
- Need for real-time insights and decision-making
- Desire for greater control over data and analytics processes
Impact on Professional Analytics Firms
The growth of in-house analytics capabilities has forced professional business data analytics firms to evolve their service offerings. While the demand for external expertise remains, these firms must now provide more specialized and value-added services to remain relevant.
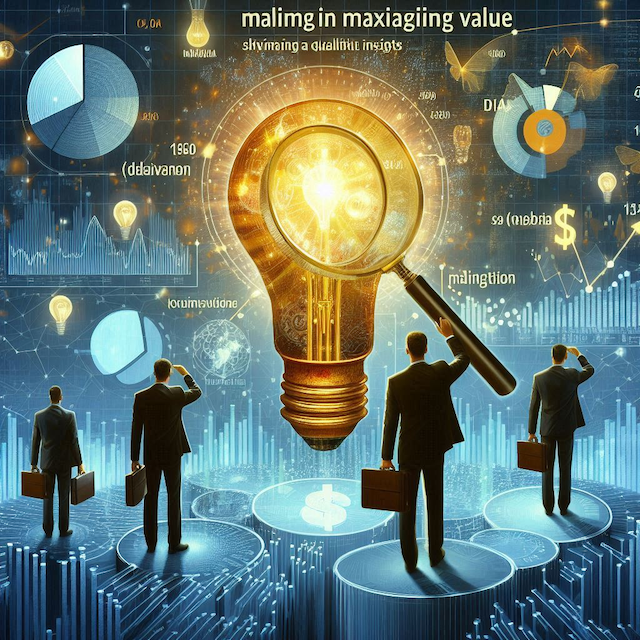
Adapting to Changing Client Needs
Expanding Service Offerings
To meet the evolving needs of clients, professional analytics firms are broadening their services to include:
- Collaborative research projects with academics and students
- Executive education and training programs
- Data strategy consulting
- Custom analytics tool development
- Data governance and compliance advisory
Case Study: Wharton Customer Analytics Initiative (WCAI)
WCAI has successfully adapted to changing market demands by:
- Offering executive education programs
- Facilitating partnerships between businesses and academic researchers
- Providing a platform for knowledge sharing and networking among analytics professionals
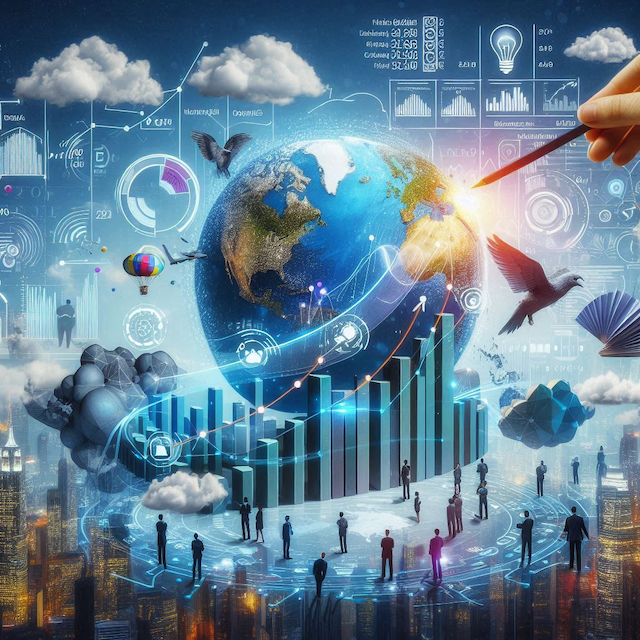
The Growing Importance of Soft Skills in Data Analytics
The Ideal Data Professional Profile
Today’s data professionals need a unique combination of skills:
- Technical expertise in data analysis and statistics
- Business acumen and industry knowledge
- Strong communication and storytelling abilities
- Project management and leadership skills
Addressing the Skills Gap
To address the challenge of finding individuals with this diverse skill set, organizations like WCAI are developing targeted education programs. These initiatives focus on:
- Teaching executives how to build and manage effective data teams
- Developing well-rounded data professionals who can bridge the gap between technical and business functions
- Fostering a data-driven culture within organizations
Breaking Down Organizational Silos with Analytics
Cross-Functional Data Applications
Data analytics is no longer confined to specific departments. Instead, it’s becoming a tool for breaking down silos and fostering collaboration across organizations. Examples include:
- Marketing and Finance collaborating on customer lifetime value analysis
- Operations and Sales working together on demand forecasting
- HR and IT partnering on employee productivity analytics
Benefits of Cross-Functional Analytics
- Improved decision-making through diverse perspectives
- Enhanced organizational alignment
- More efficient resource allocation
- Increased innovation and problem-solving capabilities
The Role of AI and Machine Learning in Modern Analytics
Augmenting Human Capabilities
While AI and machine learning are revolutionizing data analysis, human expertise remains crucial. The ideal approach combines:
- AI-powered data processing and pattern recognition
- Human interpretation and contextual understanding
- Machine learning models for predictive analytics
- Human judgment for ethical considerations and complex decision-making
Balancing Automation and Human Insight
Aspect | AI/ML Role | Human Role |
---|---|---|
Data Processing | High-speed analysis of large datasets | Defining relevant data points and metrics |
Pattern Recognition | Identifying trends and correlations | Interpreting patterns in business context |
Predictive Modeling | Generating forecasts based on historical data | Fine-tuning models and assessing real-world applicability |
Decision-Making | Providing data-driven recommendations | Making final decisions considering broader factors |
Addressing Privacy Concerns in Data Collection
The Data Privacy Landscape
As companies strive to collect more valuable data, they must navigate an increasingly complex privacy landscape. Key considerations include:
- Compliance with regulations like GDPR, CCPA, and emerging laws
- Building and maintaining customer trust
- Balancing personalization with privacy
- Implementing robust data security measures
Best Practices for Ethical Data Collection
- Transparent data collection policies
- Opt-in consent for data usage
- Regular privacy impact assessments
- Data minimization and purpose limitation
- Anonymization and pseudonymization techniques
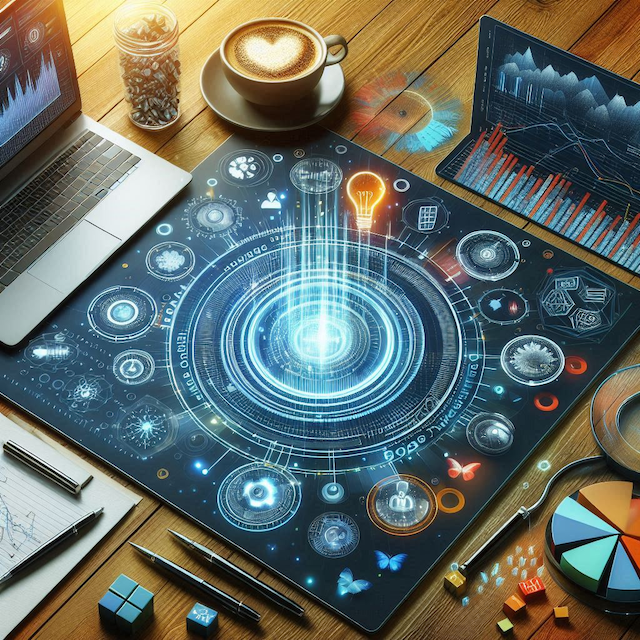
Future Trends in Customer Analytics
Emerging Focus Areas
- Predictive behavior modeling
- Real-time analytics for instant decision-making
- Integration of unstructured data (social media, customer reviews)
- Location-based analytics for personalized marketing
- Voice and image analytics
The Rise of Edge Analytics
Edge analytics, which involves processing data closer to its source, is gaining traction due to:
- Reduced latency for real-time insights
- Enhanced data privacy and security
- Lower data transmission costs
- Improved scalability for IoT applications
Conclusion
The field of business data analytics is at a pivotal juncture, transitioning from a focus on big data to an emphasis on quality insights. Professional analytics firms are adapting to this shift by offering more diverse and specialized services, while organizations are developing in-house capabilities to leverage data effectively.
The key to success in this new era lies in finding the right balance between technical expertise and soft skills, leveraging AI and machine learning while maintaining human oversight, and prioritizing data quality and privacy. As we look to the future, the ability to extract actionable insights from data will be a critical differentiator for businesses across all industries.
FAQ
Q1: How has the role of professional business data analytics firms changed in recent years?
A1: These firms have evolved from primarily offering outsourced analytics services to providing a broader range of offerings, including collaborative research, executive education, and specialized consulting services.
Q2: What is the difference between big data and quality data?
A2: While big data focuses on volume, velocity, and variety of information, quality data emphasizes accuracy, relevance, timeliness, consistency, and completeness of information that can drive actionable insights.
Q3: Why are soft skills becoming increasingly important in data analytics?
A3: Soft skills such as communication, business acumen, and leadership are crucial for translating technical insights into business value and fostering cross-functional collaboration within organizations.
Q4: How are companies addressing privacy concerns in data collection?
A4: Companies are implementing transparent data collection policies, obtaining opt-in consent, conducting privacy impact assessments, and using data minimization and anonymization techniques to address privacy concerns.
Q5: What are some emerging trends in customer analytics?
A5: Key trends include predictive behavior modeling, real-time analytics, integration of unstructured data, location-based analytics, and the rise of edge analytics for improved performance and privacy.